Classical forecasting tools
Click here for a short explanation
Advantages of classical forecasting tools
Advantages of these approaches are that they are simple to perform and easy to understand. The time trend deals with the data sequentiality. Heterogeneity can be captured by the extended approaches that allow for different intercepts and slopes. Volatility and non-linearities can only be dealt with in a limited way.
Auditability is very high as modelers explicitly choose the model structure and can use statistical tests to support their choices.
Extensions of this approach include the following
- Allowing for different intercepts β0i for various countries (linear panel data regression)
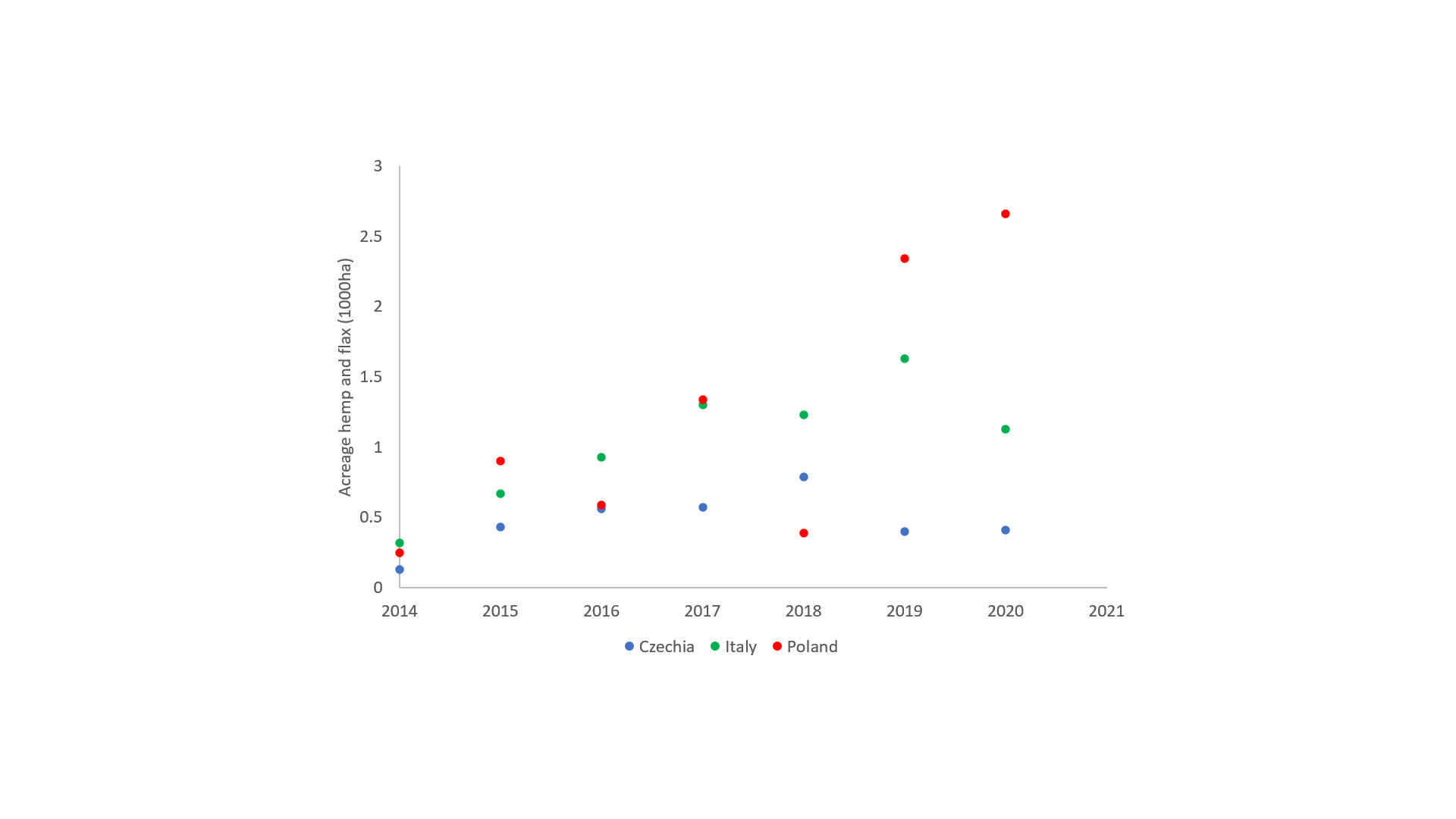
- Allowing for different slopes β1i for various countries (random coefficient model or model separately analysed for each country)
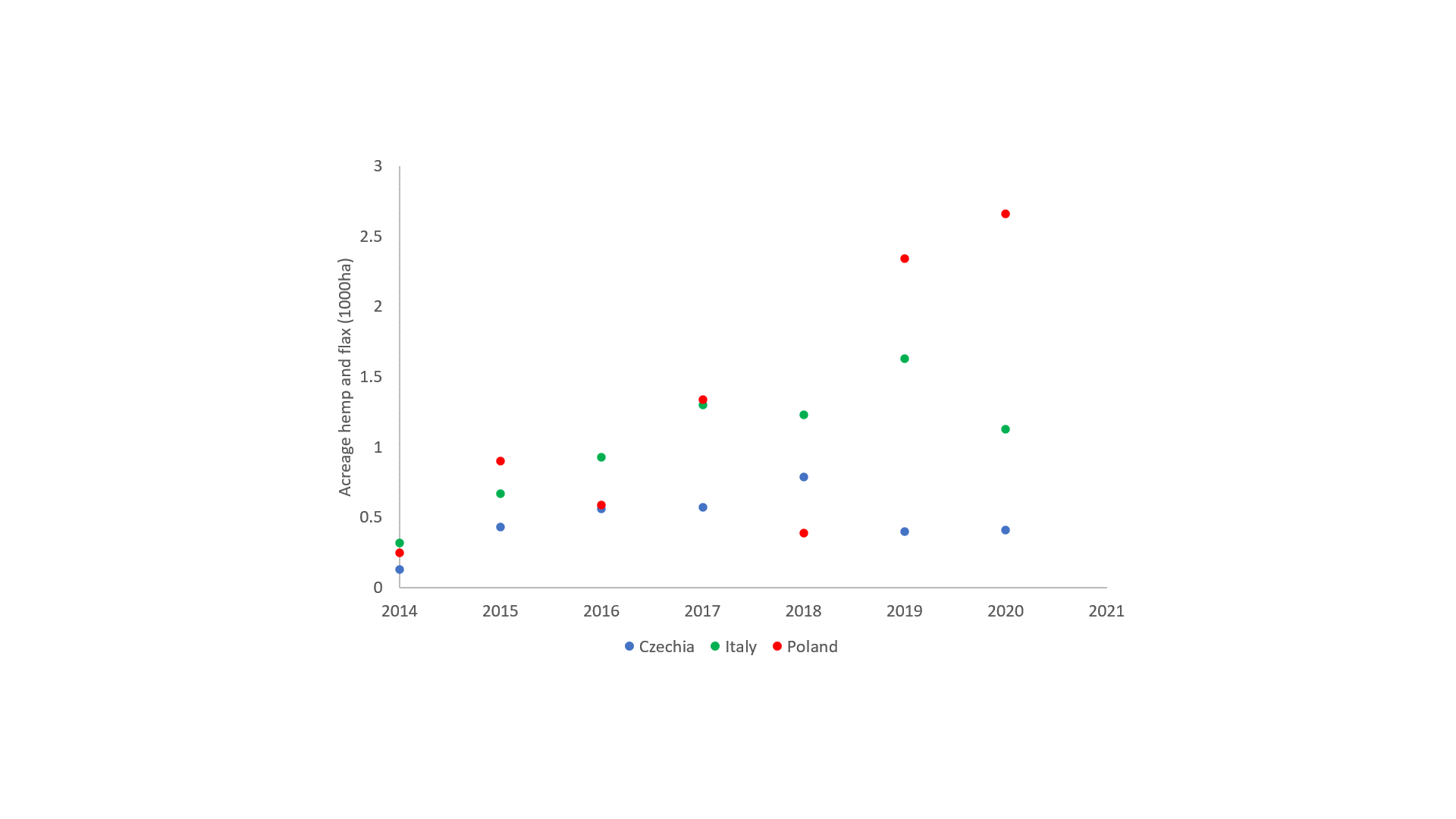
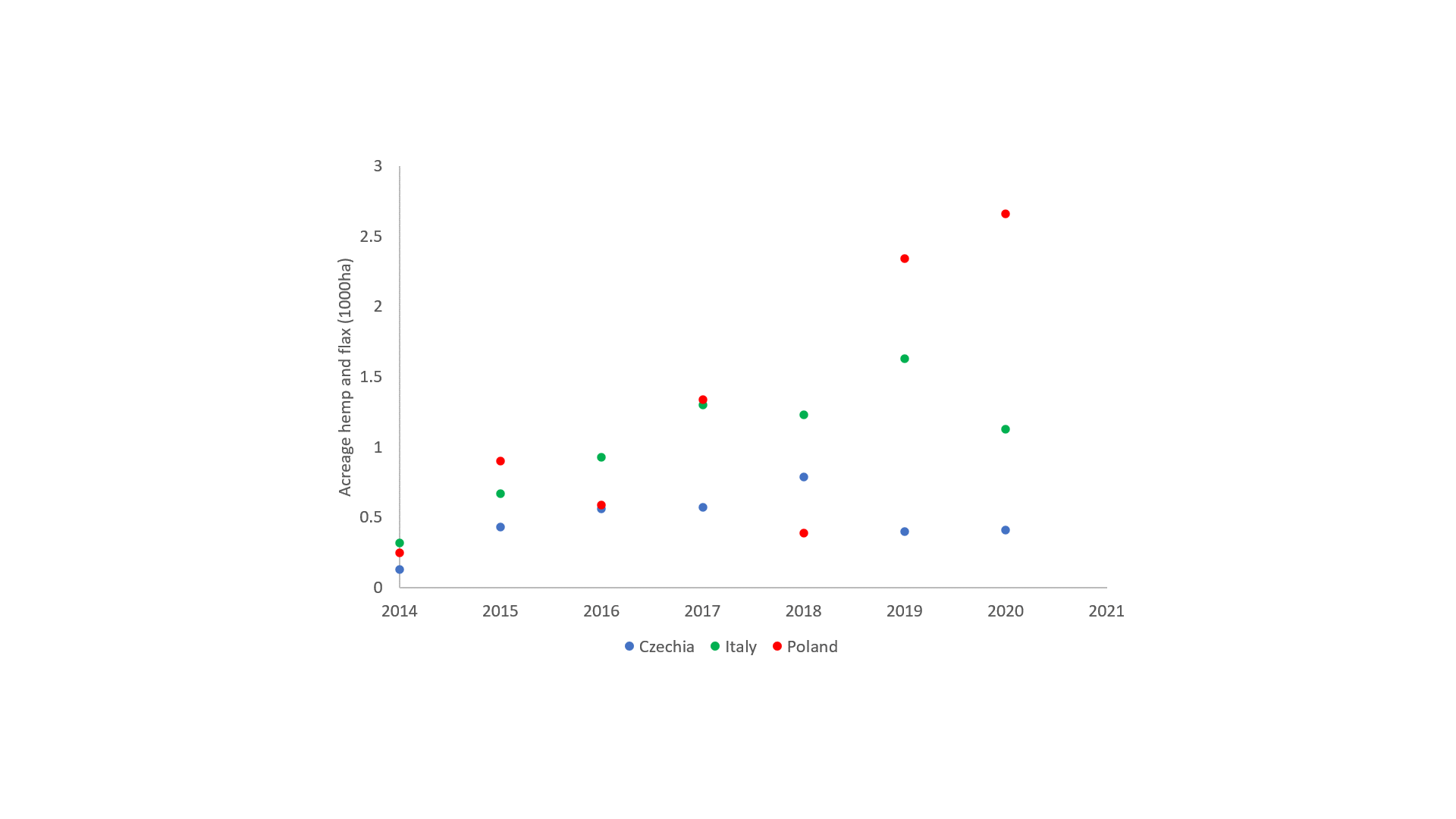
- Including drivers, such as policy variables and prices in the above models
- Allowing for quadratic and interaction terms of variables to capture non-linear patterns
Short explanation of classical forecasting tools
Classical forecasting tools can be summarized as linear trend line fitting. Simple examples are drawing or fitting simple straight lines through existing datapoints. Forecasts are based on linear extrapolation of such lines.
Statistically this could be done using linear regression in which an intercept and slope parameter of a trend variable are estimated:
acreaget=β0+β1⋅t+εt
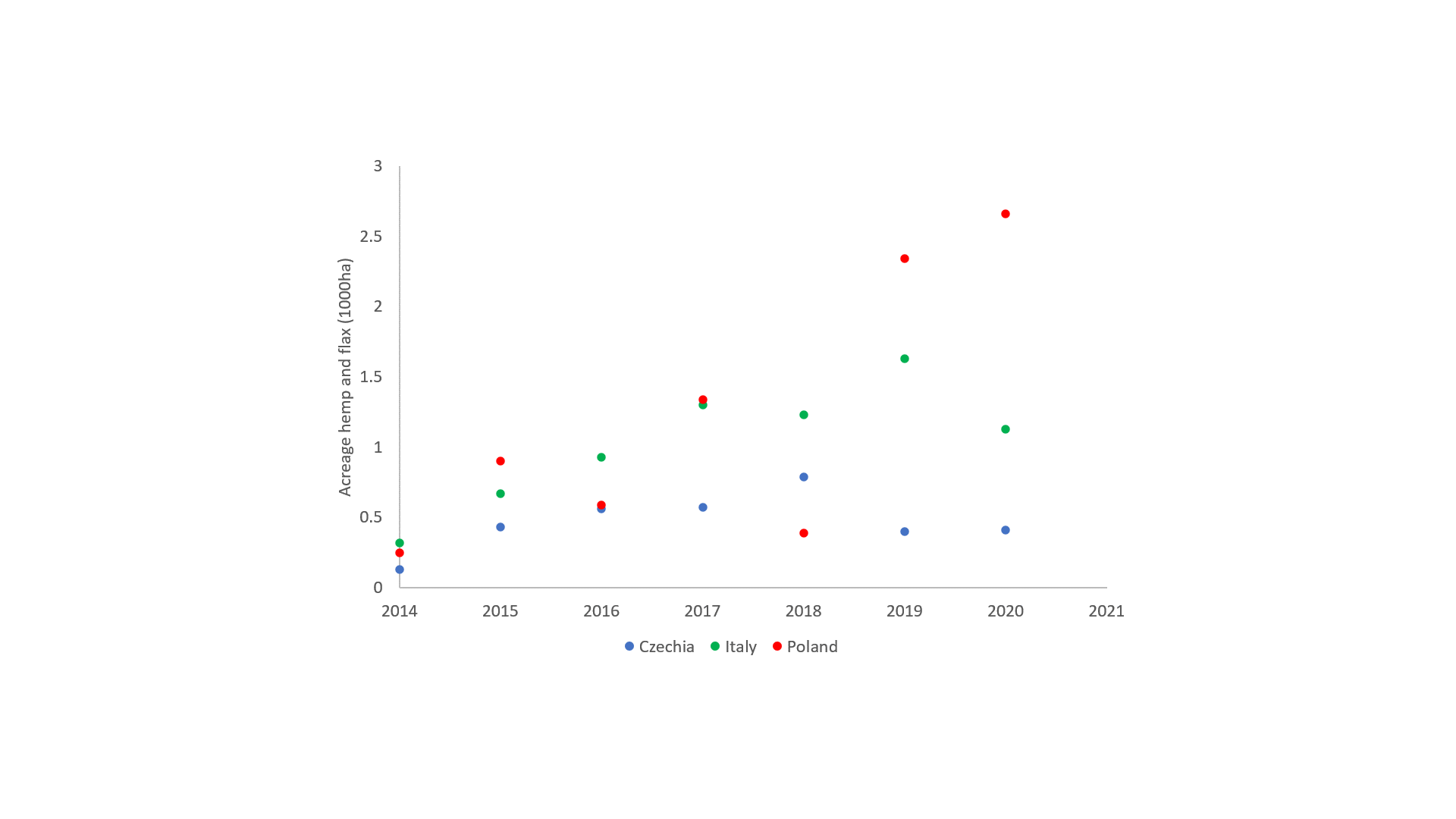